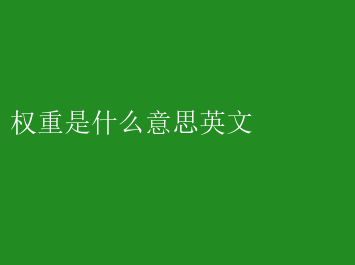
The Significance and Applications of Weights in Data Analysis andBeyond
In the vast landscape of data analytics, statistics, and machine learning, the concept of weights holds paramount importance. It serves as a pivotal tool for adjusting the influence of individual data points, enhancing the accuracy and relevance of models, and ensuring that analyses reflect the true nature of the underlying phenomenon. This article delves into the essence of weights, explores their diverse applications, and underscores their indispensable role in the world of data-driven decision-making.
Understanding the Fundamentals
At its core, a weight is a numerical value assigned to a data point or observation, indicating its relative importance or contribution to the overall analysis. These weights can be either inherent to the data(e.g., population size in survey sampling) or artificially assigned based on certain criteria(e.g., to account for missing values or outliers).
In statistical models, weights are often employed to correct for biases in the sample data or to give more emphasis to certain observations that are deemed more representative or informative. For instance, in survey research, weights can be used to adjust for non-response bias, ensuring that the results reflect the characteristics of the entire population, not just those who responded.
Applications in Data Analysis
1.Weighted Averages
One of the